Biomaterials

(i) Multi-scale modeling for property measurement of protein-inspired materials and prediction using machine learning: Through all atomistic simulations and machine learning, we have contributed predictions of both bulk mechanical properties, mesoscale structure formation, and residue-level information. Using an energy renormalization approach, we developed catechol content-specific coarse-grained models of poly(catechol-styrene) inspired by the remarkable adhesive properties of marine mussels. These models captured toughness, shear modulus, elastic modulus, and ultimate tensile strength well in comparison to all atomistic models. Later, we used all atomistic models of mussel foot protein 5 inspired biomaterials to demonstrate the outsize role of charged residues and densification effects in enhancing the strength and toughness of intrinsically disordered proteins.
Beyond modeling mechanical properties, we have also made progress in predicting residue-level information of highly ordered proteins such as Debye-Waller factor, also known as B-factor in proteins. Using experimental data collected from the Protein Data Bank, we developed a sequence-based deep learning model with the best performing B-factor prediction to date. By using a long short-term memory neural network (LSTM), we showed that the amino acid primary sequence alone gives an excellent correlation between predictions and ground truth measurements. This finding was surprising because secondary structure and atomic coordinates intuitively communicate critical orientation and spatial information of amino acids in a machine learning environment. Indeed, the inclusion of these features nearly doubled the Pearson correlation coefficient (PCC) of a simple recurrent neural network, but only improved the PCC of the LSTM by a few percent. This finding emphasized the importance of LSTM in diminishing the vanishing gradient problem present in learning information about long proteins and highlighted the astounding amount of information that can be extracted from the primary sequence.
Beyond modeling mechanical properties, we have also made progress in predicting residue-level information of highly ordered proteins such as Debye-Waller factor, also known as B-factor in proteins. Using experimental data collected from the Protein Data Bank, we developed a sequence-based deep learning model with the best performing B-factor prediction to date. By using a long short-term memory neural network (LSTM), we showed that the amino acid primary sequence alone gives an excellent correlation between predictions and ground truth measurements. This finding was surprising because secondary structure and atomic coordinates intuitively communicate critical orientation and spatial information of amino acids in a machine learning environment. Indeed, the inclusion of these features nearly doubled the Pearson correlation coefficient (PCC) of a simple recurrent neural network, but only improved the PCC of the LSTM by a few percent. This finding emphasized the importance of LSTM in diminishing the vanishing gradient problem present in learning information about long proteins and highlighted the astounding amount of information that can be extracted from the primary sequence.
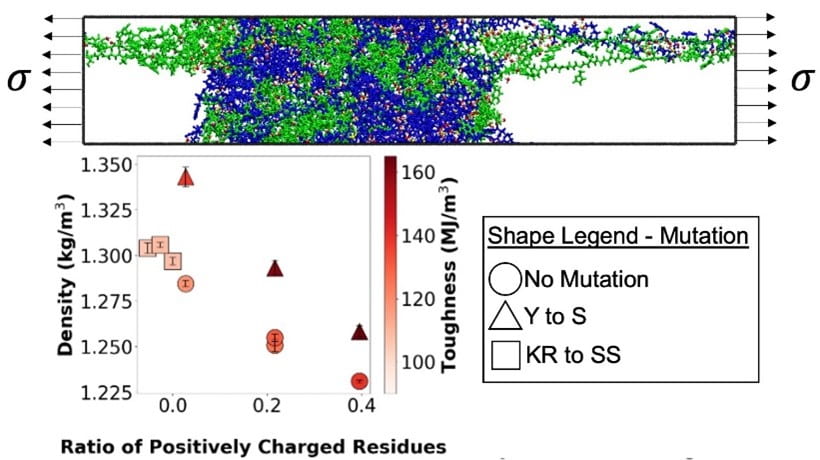
Fig. Toughness correlates positively with mutation-induced changes in charge and density. (Graham, Keten ACS Biomaterials Science and Engineering, 2023)
We have developed a combination of simulation and data-driven approaches to explain the relationship between sequence, processing, and property of synthetically produced protein-based fibers. Mesoscale features of assembled proteins such as amorphous matrix encapsulated beta crystals characteristic of spider silk critically impact mechanical performance. Many such features are tunable through the manipulation of processing conditions and primary sequences. This opens the possibility for fibers with modular mechanical properties. Using a dissipative particle dynamics model, we have shown that flow-induced crystallization and network formation during fiber spinning varies between shear and elongational flows which is a tunable processing parameter. To elucidate sequence effects, we have used machine learning approaches to identify sequence features that correlate with mechanical properties of both spider-silk based fibers and fibers spun from titin immunoglobular domains 67-70. This work is aligned with our group’s ‘materials-by-design’ approach by which experimental data, simulation, and machine learning are coordinated to develop materials for advanced applications like therapeutics, packaging, and warfighter protection.
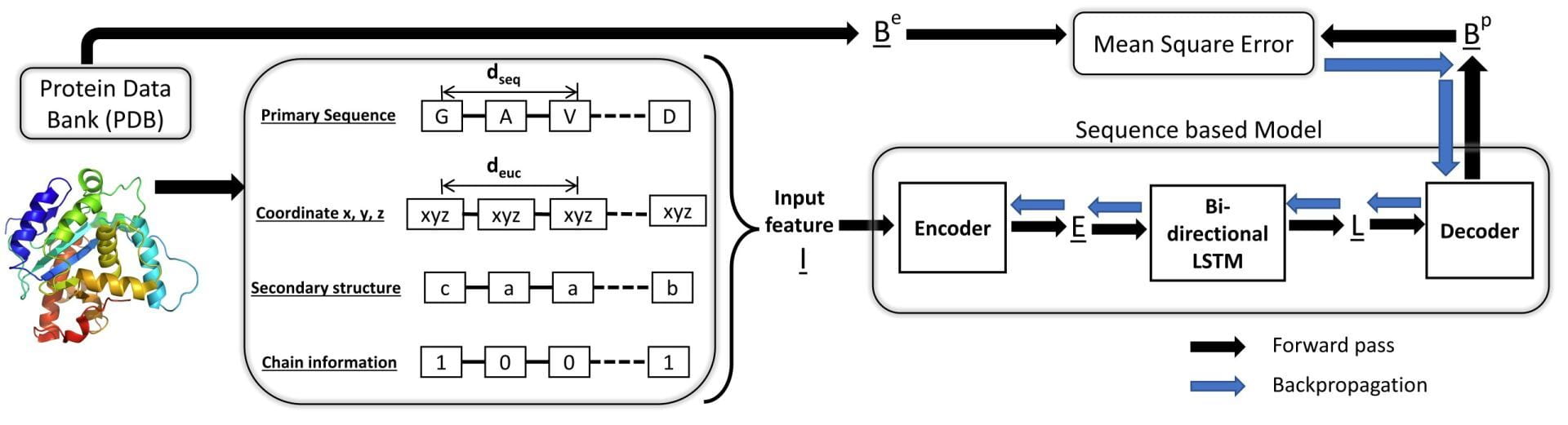
Fig. Deep learning model architecture for B-factor prediction. (Pandey et al. Patterns, 2023)